Responsible AI: From vision to value
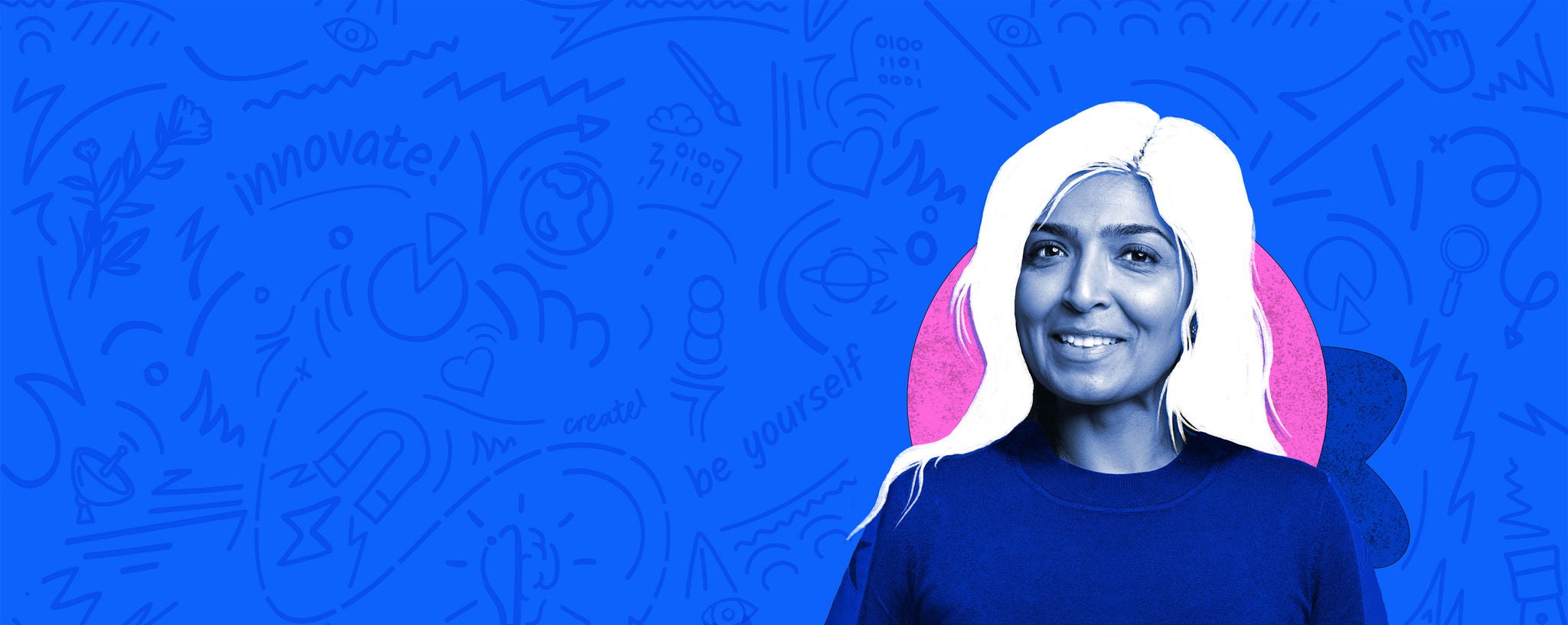
Artificial intelligence (AI) is quickly becoming accessible to everyone, whether through microfinancing apps in developing countries or connecting with friends and family on social media.
However, early adopters of AI are discovering that it’s difficult to fully understand the risks and the impact of bias or misuse. The results can be massive, as we saw mid-2020 in the UK with the “F*** the algorithm” protests and with Silicon Valley tech giants who are often in the press and courts for pushing the limits of ethical and compliant machine learning (ML). Leading organisations are those that accept and manage the risk of AI while striving for responsible AI by design.
Why it’s good for your business
Let’s look at how responsible AI translates to business value. Historically, ethically driven behaviour as in “doing the right thing” has been seen as either an overhead cost or merely an exercise in public relations building—lacking obvious value for the business. The question is now, How does doing the right thing also make good business sense? If trusted organisations outperform less trusted counterparts by 300%, how do we start to instrument and measure this?
A successful organisation will strike the balance between value for itself and benefits for its customers, while avoiding customer exploitation. Consider an insurance company that scrapes Facebook to predict how likely an individual is to make a claim in the future. Even if this behaviour wasn’t in breach of social media guidelines, a small and short-term advantage would be quickly wiped out once the tracking became public knowledge.
Responsible AI can help your business by:
- Building trusted customer relationships
When customers trust a brand, they’re more likely to be loyal to it and interact with less cynicism. Trust in this case can be generalised as the company’s ability to provide a great product or service in a reliable and transparent manner, while avoiding self-orientation (i.e., having only the company’s interest in mind). Trust leads to companies having better retention, spend, and adoption of new services. - Creating employee evangelism
More than ever before, employees want to work for a purpose-driven company. Whereas 20 years ago, financial remuneration was the dominant driver in recruiting new talent, 64% of millennials today won’t take a job if a company doesn’t have strong corporate social responsibility values—and 83% would be more loyal to a company that helps them contribute to social and environmental issues. - Differentiating with proactive compliance readiness
With ever-increasing government and industry regulations—as well as customers’ rising expectations for fairness and privacy—proactive and early adoption can be a differentiator. Many companies worldwide adopted aspects of the EU-focused General Data Protection Regulation (GDPR) even if they didn’t expect near-term direct applicability. - Increasing brand approval
Users are open to interacting with intelligent apps and services, in some cases with obvious AI such as a chatbot or a call router, but they’re also quick to strike back against even the smallest signs of mistrust, bias, or unapproved behaviours. Some brands have recently tried to show their prioritization of privacy and trust, like Apple’s public support of transparent AI and strong data privacy and controls.
Whatever your role, here are some quick ideas to get started.
Five steps to realising value with responsible AI
Step 1: Build a diverse team
When we’re designing AI solutions, we’re less likely to make mistakes when an idea is fully interrogated by a diverse set of stakeholders. Google and other AI leaders have discovered the importance of building diverse teams to minimize racial, gender, and other forms of bias in AI.
Neurodiversity involves bringing together technical and data specialists who can build the technology, experience and emotional intelligence specialists who understand the human impact, business leaders who know the value, and legal and compliance.
MIT recently shared a story of creating leading text analytics by pairing linguistic experts with data scientists—bringing their diverse skills together to create a less biased AI.
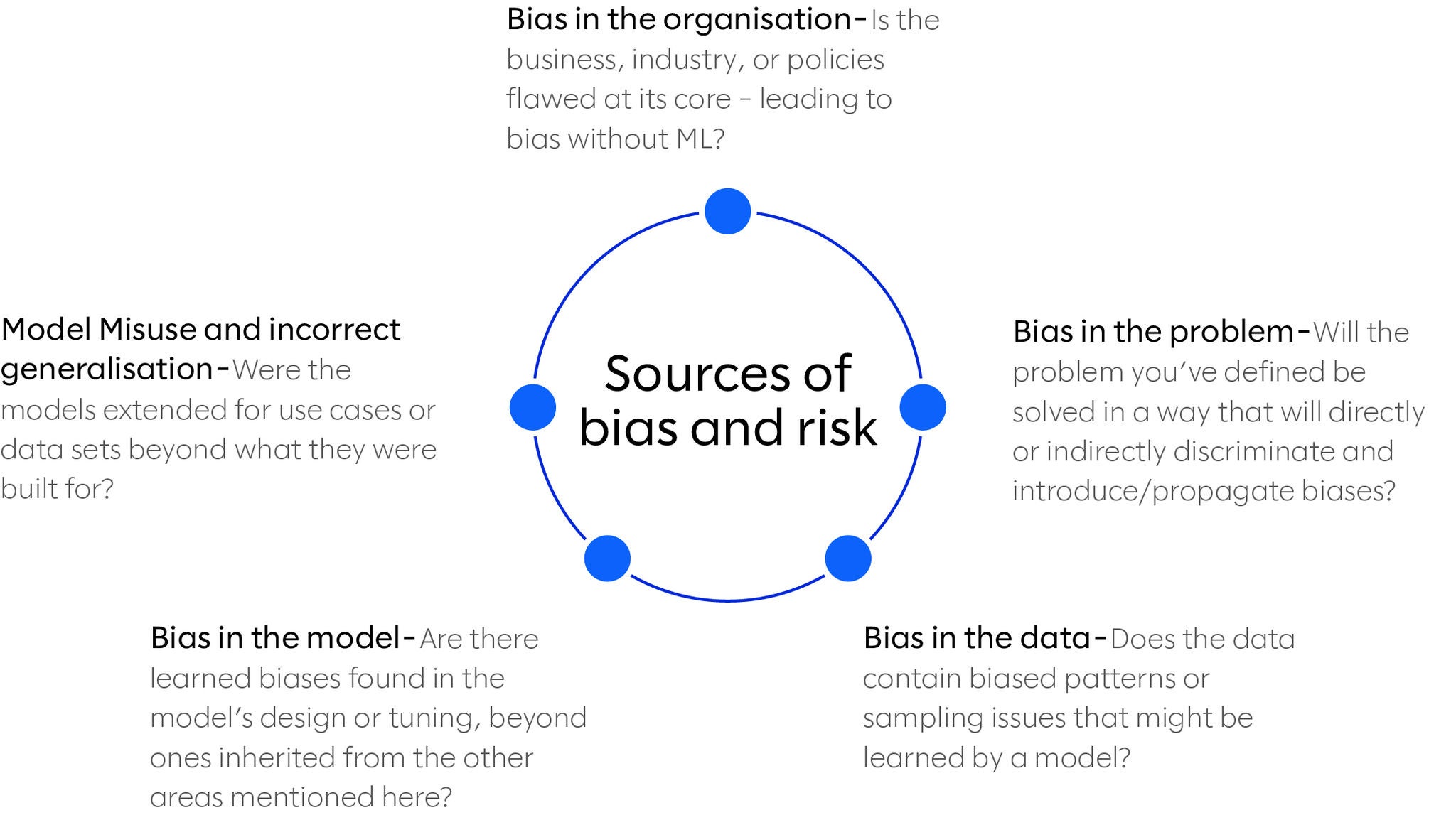
Step 2: Humanise the complexity
Deploying your first AI solution may seem overwhelming, like there are insurmountable risks and challenges. We recommend taking a stern look at the complexity and risks but being pragmatic with how you manage them. Most importantly, put the human perspective back into the problem where possible. After all, ethics and fairness are normally defined by culture and communities rather than hard science. In some cases, this may mean reducing the autonomy or scale of the AI solution after factoring in the human point of view, which may hint at hidden risks.
We see this dilemma appear in drug development and treatment with the risk of “paternalistic algorithms,” which are optimised to deliver the single, subjective outcome of increasing the duration of a patient’s life, without considering the quality of life. It’s important to be able to clearly articulate a position on trade-offs like these before ML models get to work.
In some cases where AI works in real time, organisations have created risk management functions to complement the AI initiatives and identify, handle, and retrain the AI cases that aren’t handled well. Following criticism over its power to censor and the unclear influence of its AI on laws and ethics, Facebook created a board of 19 individuals to adjudicate over complaints or hot topics, most recently whether Donald Trump’s account should be unfrozen. Human experts are well suited for adjudicating over these complex topics and can work with the AI to filter out improper posts and images.
Step 3: Augment, don’t replace, humans
Going a step further than using humans to problem-solve when necessary, put humans at the centre of the solution via “augmented AI” rather than traditional autonomous and independent AI. For example, Slalom worked with pathologists from multiple research organisations, including the American Cancer Society, to use AI to do the time-intensive task of screening images to flag the high-risk ones with tumour cells. This allows the human experts to focus on diagnosing and collaborating on triaged findings—and to even identify high-risk cells earlier than ever before.
Another example is in the UK, where the National Health Service uncovered systematic prescription fraud among dentists. Tackling this problem was a neurodiverse team of engaged business leads, enthusiastic data science PhDs, and experienced, grounded IT and data analytics veterans working together. After weighing potential patient impacts and regulations, the team designed for an augmented AI approach, where AI complements rather than replaces humans. Trawling more than 90 million prescriptions per month was not a job that existed, but AI’s unique ability to process dull, detailed, and high-volume workloads like this meant that potential cases could quickly and autonomously surface to human investigators.
Step 4: Balance risk with measured controls
How do you predict, manage, and mitigate critical risks while maximising the return of investments for your business and customers?
One way is to assign a risk level for the AI use case based on something like financial, brand, life, or regulation impact against frequency or probability of occurrence. For example, when a recommendation engine makes a product suggestion—a frequent occurrence—and it’s a poor suggestion, it’s ultimately inconsequential. At the other extreme, an unsuccessful prosecution of an alleged murderer based on AI-derived evidence is far more damaging but does not operate at anywhere near the same levels of volume and real-time latency at which the recommender example occurs.
It’s also critical to make sure an application with ethical intent can achieve this universally or be restricted to the context it was strictly designed for. For instance, a smartwatch app which monitors things like an individual’s speech pattern and heart rate—and vibrates when “the user is tired”—can arguably be viewed as benign and helpful. But if it’s used by insurance companies to identify early signs of neurological diseases or to generalise users into fitness segments, then there’s potential for nefarious and unethical results.
Creating guardrails for the teams to explore AI will result in a sense of freedom and awareness of what’s permissible. We’ve seen over the years that an ultra-conservative approach will stifle innovation, and in many cases, it’s much too severe for the use case.
Step 5: Monitor closely and challenge often
AI is advancing quicker than governments and industries are developing ethical regulations on how to use it. Because of this, each organisation is responsible for measuring the potential success versus risks of an AI solution and deciding what is, and isn’t, ethical. How do you do this?
You need to break down the core elements of trust and ethics—defined by the United Nations as benevolence, integrity, and the capability to create something meaningful—into a quantifiable, measurable framework. However, the world is in the early days of formalising the ethics of AI, and organisations will need to proactively adopt early frameworks and best practices from other organisations. Some examples are open sources and commercial tools such as TruEra and AWS and academic frameworks from CMU and Harvard.
We also recommend reading this University of Oxford article on the Right to Reasonable Inferences as a primer on which social expectations could quickly become legal obligations for businesses using AI in terms of the rights of someone on the other end of the AI.
The power only humans hold
AI has huge potential to change the world for better or worse. But it’s still far from having the human superpowers of morality, empathy, and soul. Anyone hearing the songs of an AI algorithm will attest to the latter! It’s by keeping real people with diverse perspectives involved in the decision-making process that will unlock truly positive, ethical, and trusted use cases for AI.
To quote University of Oxford’s data ethics committee: it’s up to us.