AI in pharma: Tech titan or hype train?
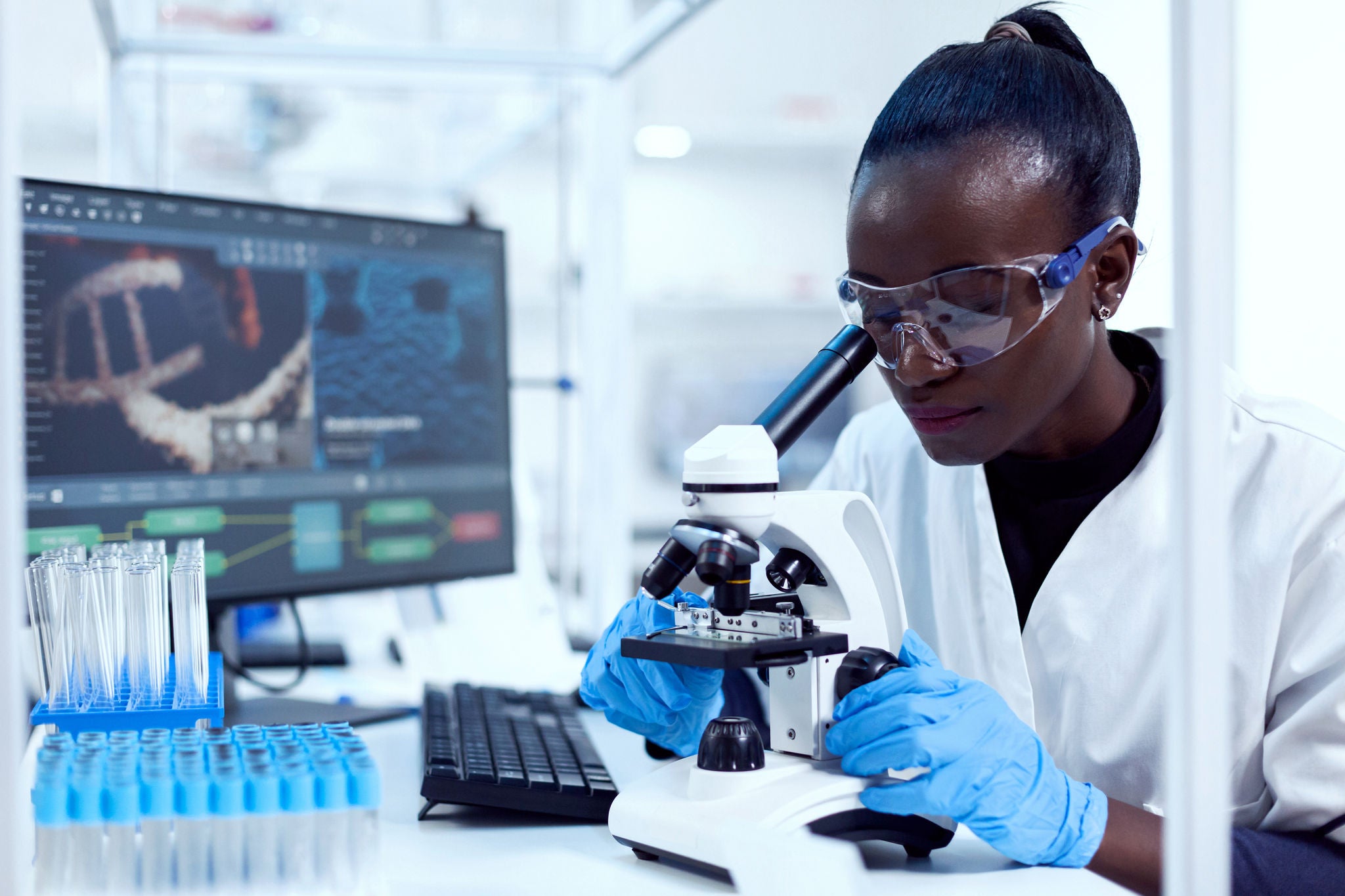
Over the past decade, pharmaceutical companies have undergone significant transformations driven by more progressive regulations due to advancements in manufacturing control processes and automation technology. This shift has led to increased efforts in innovation and investment in new technologies within the pharmaceutical industry. The primary goal behind this transformation is to ensure a more consistent quality of products, ultimately enhancing patient safety.
Interestingly, the traditional pharmaceutical giants are not at the forefront of this change. Instead, the emerging field of cell and gene therapies (CGT) is leading the charge. The processes involved in CGT are incredibly complex and dynamic, requiring a heavy reliance on automation and digitization to meet the desired quality and performance attributes of drug products. This shift toward automation and digitization has been fueled by therapeutic demand and a technological revolution within the industry.
Emergence of generative AI
Generative AI (GenAI) has garnered significant attention within the pharmaceutical and healthcare sectors. Since the launch of ChatGPT in 2022, GenAI has dominated headlines, sparking intense competition among businesses wanting to harness its potential value. GenAI holds promise in various areas of the CGT industry, from accelerating drug discovery to enabling personalized treatments, optimizing clinical trials, and enhancing bioprocessing, among others. However, the pharmaceutical industry is no stranger to buzzwords and tech-driven promises—remember blockchain?
Comparing the hype: Blockchain vs. GenAI
Drawing parallels between the blockchain hype cycle and the excitement surrounding GenAI is not unwarranted. Blockchain, which initially disrupted the financial world with promises of decentralization, transparency, data storage, and traceability, eventually faced challenges during its implementation across various industries. As the dust settled and pharmaceutical companies came to realize the full integration of blockchain into existing systems, updating processes to support adaption efforts while adhering to stringent regulatory requirements demanded a level of maturity that most were not ready for. Similarly, GenAI is currently surrounded by high expectations, and skeptics are not too far-fetched to question its lasting value, considering its true application is still a mystery to many. As GenAI emerges as a disruptive force, stakeholders must heed the lessons learned from blockchain as valuable insights.
For example, blockchain was slated to revolutionize data management and collaboration, but many drug development use cases pushed the technology’s limitations. Despite its appeal and claims of data integrity and security, blockchain stumbled over issues like scalability, interoperability, and data privacy, and it fell short when it came to enabling teamwork and knowledge sharing.
Blockchain promised transparency, supply chain efficiency, and quality control for manufacturing. However, manufacturers quickly learned that integrating blockchain into their operations demanded careful planning, hefty investment, and adaptability.
Compliance also emerged as a significant challenge during the integration of blockchain technology. The decentralized nature of blockchain brought a whole new set of regulatory headaches, leaving manufacturers questioning how to adopt the technology. Early attempts to navigate compliance issues underscored the importance of establishing clear regulatory frameworks and standards tailored to blockchain applications.
While blockchain and GenAI share similarities in disruptive potential, they also have fundamental distinctions essential to understanding their implications. A report from Mastercard states, “Unlike other technologies that have seen hype cycles, generative AI exhibits clear use cases, has led to the creation of robust solutions, and is developing swiftly.” The report also contends that GenAI is poised to be transformative across nearly every sector. However, skeptics of GenAI raise valid concerns. Current GenAI platforms lack a deep understanding of the intricate biological systems involved in CGT research and manufacturing. While proficient at data processing and pattern recognition, GenAI struggles with interpreting the subtleties of biological processes. Scientific research is highly unpredictable, requiring constant adaptations and creative solutions—areas where GenAI falls short. CGT operates within a complex ethical and regulatory framework, necessitating human judgment and moral considerations.
Developing true GenAI capable of overcoming these challenges is a monumental task that may take years to accomplish. As we await the next technological evolution, there are valuable lessons to be learned from the blockchain hype that can guide our approach to GenAI. Both blockchain and GenAI are versatile digital technologies with a wide range of potential applications. It is crucial to thoroughly assess their features and apply them when they are the most suitable solution for a specific problem. This assessment should also compare GenAI to alternative technologies to determine its best fit.
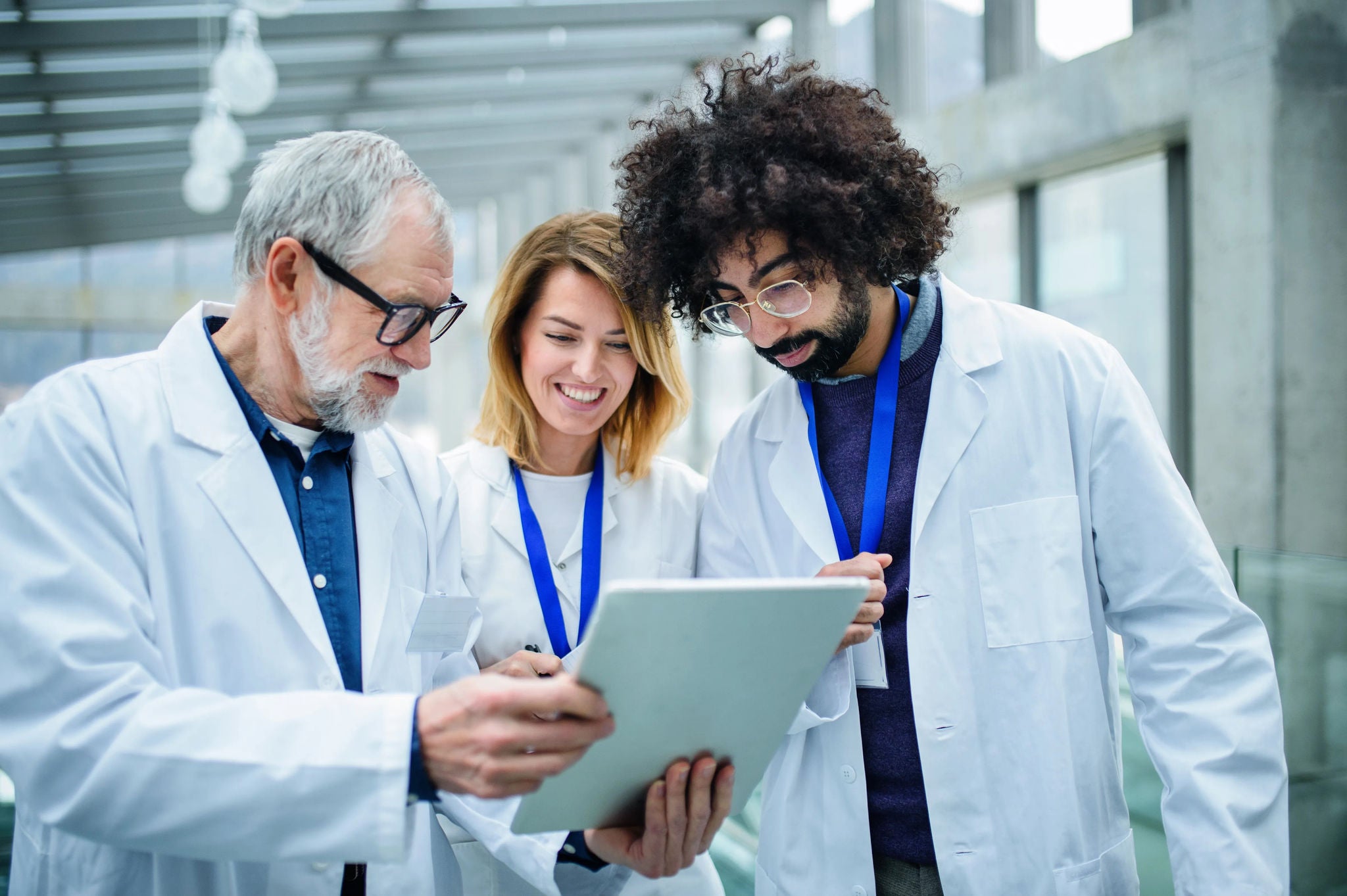
Integration of GenAI into the CGT industry
To effectively integrate GenAI into the healthcare sector, especially within CGT, it must be viewed as a complementary addition to existing systems and technologies. CGT companies should encourage a gradual, controlled integration of GenAI that enhances and complements the current healthcare ecosystem. Incremental testing and evaluation are essential steps to mitigate potential risks associated with its implementation. Moreover, educating stakeholders, including patients and the public, about GenAI’s capabilities and implications for data ownership, access, and privacy is crucial. GenAI applications should prioritize user-centric design principles to ensure they are user-friendly and meet the needs of both healthcare providers and patients.
A critical challenge of integrating AI and GenAI is data dependency. Curating high-quality datasets enhances the reliability and accuracy of AI-generated insights. Organizations should ensure ethical AI implementation through robust frameworks that consider the complex ethical and regulatory framework within which CGT operates.
Given the unique features of GenAI, compliance with existing laws, regulations, and data governance frameworks is paramount. Recently, the FDA published two documents—one on the use of AI and machine learning in medical devices and another on the development of drug and biological products — indicating their increasing openness to AI-driven technologies in healthcare and recognizing the potential to transform the industry. The Digital Health Innovation Action Plan highlights the FDA’s commitment to modernizing regulatory pathways for digital health tools, such as IoT, blockchain, and AI applications.
In the grand scheme of things, blockchain and GenAI are distinct phenomena, each with its own challenges and opportunities. Recognizing their strengths, limitations, and tangible merits is essential to determining their value proposition within their respective domains. While GenAI holds great promise for the future of healthcare and pharmaceuticals, it must be approached with caution, acknowledging its current limitations and the need for human expertise.
Partnering for success in the future of medicine
Engaging a partner with regulatory and quality expertise can guide companies through the total product life cycle to promote an industry best-in-practice approach to compliance and accelerate the marketing authorization approval processes.
In this evolving landscape, Slalom can offer a transformative tool kit for gene therapy manufacturing. We leverage digital strategy, analytics, and innovation to optimize processes, adapt to the dynamic challenges in the gene therapy field, and unlock the full potential of GenAI—leading to groundbreaking therapies, improved patient outcomes, and revolutionized healthcare. Our data scientists and AI experts develop interpretability frameworks that bridge the gap between complex AI-generated outcomes and actionable insights. These frameworks enable researchers and clinicians to make informed decisions based on AI insights, translating them into understandable and usable information.
As the pharmaceutical and healthcare industries evolve, strategic partnerships with organizations like Slalom can play a pivotal role in shaping the future of medicine and patient care.