AIOps: A holistic approach to operationalizing AI
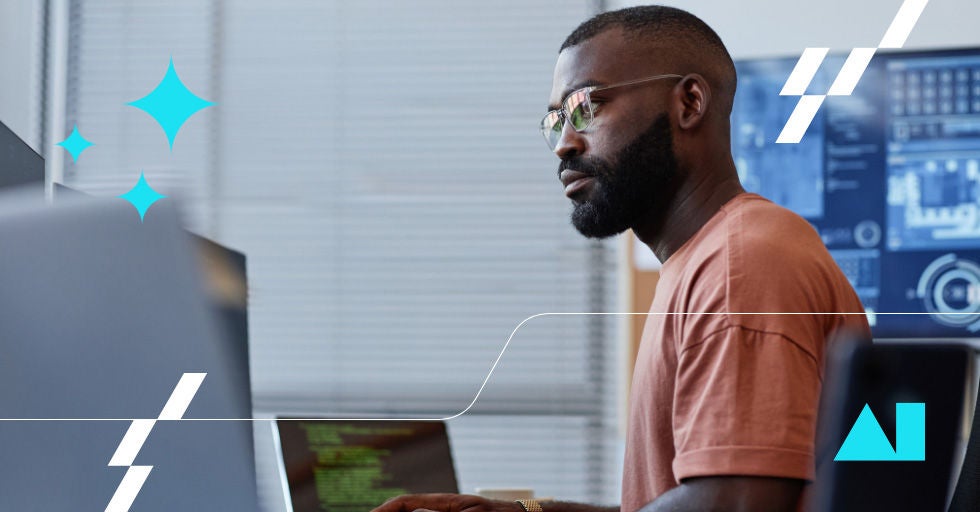
In recent years, AI has moved from the fringes of innovation to the heart of business strategy. The explosion of generative AI (GenAI) in 2023 marked a turning point, where businesses began to see AI not just as a tool for experimentation, but as a transformative technology capable of driving significant business value. This ongoing shift is reflected in our research, with most organizations—82%—planning on increasing their AI spending in 2025. However, despite the enthusiasm and investment, many organizations struggle to transition from proof of concept (POC) to full-scale production. Peter Bendor-Samuel, CEO of research firm Everest Group, projects that 90% of AI POC pilots won’t reach production in the near term, with some never making that leap.
As AI has advanced, so have the operational frameworks needed to support it. Artificial intelligence operations (AIOps) enables businesses to systematically integrate AI and machine learning (ML) capabilities across their operations. Achieving this integration efficiently and effectively requires more than just deploying technology; it demands a holistic, human-centered approach. As businesses continue to integrate AI into their products and operations, it’s essential to manage the complexities, establish a strong foundation, and maintain discipline to ensure AI initiatives deliver real value.
Getting stuck: Why AI falls short of its potential
Despite the transformative potential of AI, many organizations still face significant challenges in operationalizing it effectively, particularly when moving from experimentation to scalable, real-world applications. One of the most common issues is the lack of connection between AI-generated insights and a meaningful, user-centric experience that turns those insights into actionable outcomes. Without an intuitive, impactful experience, even the most powerful AI solutions may struggle to drive real-world change.
Common barriers that prevent AI from delivering its full potential include:
- Risk aversion: Many organizations hesitate to experiment with AI due to real concerns about navigating regulatory, security, and ethical challenges. In turn, they often struggle to turn this risk aversion into actionable, statistically sound testing frameworks and automated feedback mechanisms that could help mitigate these risks. Without these tools, organizations remain cautious, limiting AI’s potential to drive innovation.
- The POC trap: Many businesses are unable to transition from POC to production. This results in wasted resources and missed opportunities as AI initiatives fail to scale or integrate into broader business strategies.
- Scalability challenges: Managing and deploying multiple AI models at scale requires careful planning. Systems not designed with scalability in mind struggle to integrate, maintain, or adapt to growing demands, leading to inefficiencies and increased costs.
Collectively these challenges hinder organizations from fully operationalizing AI. This is where a unified AIOps framework becomes key, addressing these issues head-on by ensuring seamless model deployment, ongoing monitoring, and rapid scalability.
Moving forward with AIOps
AIOps is the critical practice of AI engineering focused on operationalizing the full lifecycle of AI-powered systems and assets. It ensures that AI models move seamlessly from development to production, emphasizing testing and statistical validation, deployment, monitoring, and continuous optimization. AIOps builds on the foundations of machine learning operations (MLOps), offering the flexibility to operationalize all AI assets responsibly and efficiently.
AIOps requires a set of tools, or architecture patterns, that work together to address various operational challenges. While many of these tools share a common foundation—using the same data—different use cases require different approaches. Some situations can be handled with the same core tools, while others demand a broader or more specialized set of solutions. Over time, as the AIOps platform evolves, it becomes more versatile and capable of supporting a wider range of use cases. Depending on the complexity of their operations, companies may need anywhere from two to 10 tools or architecture patterns to meet their specific needs.
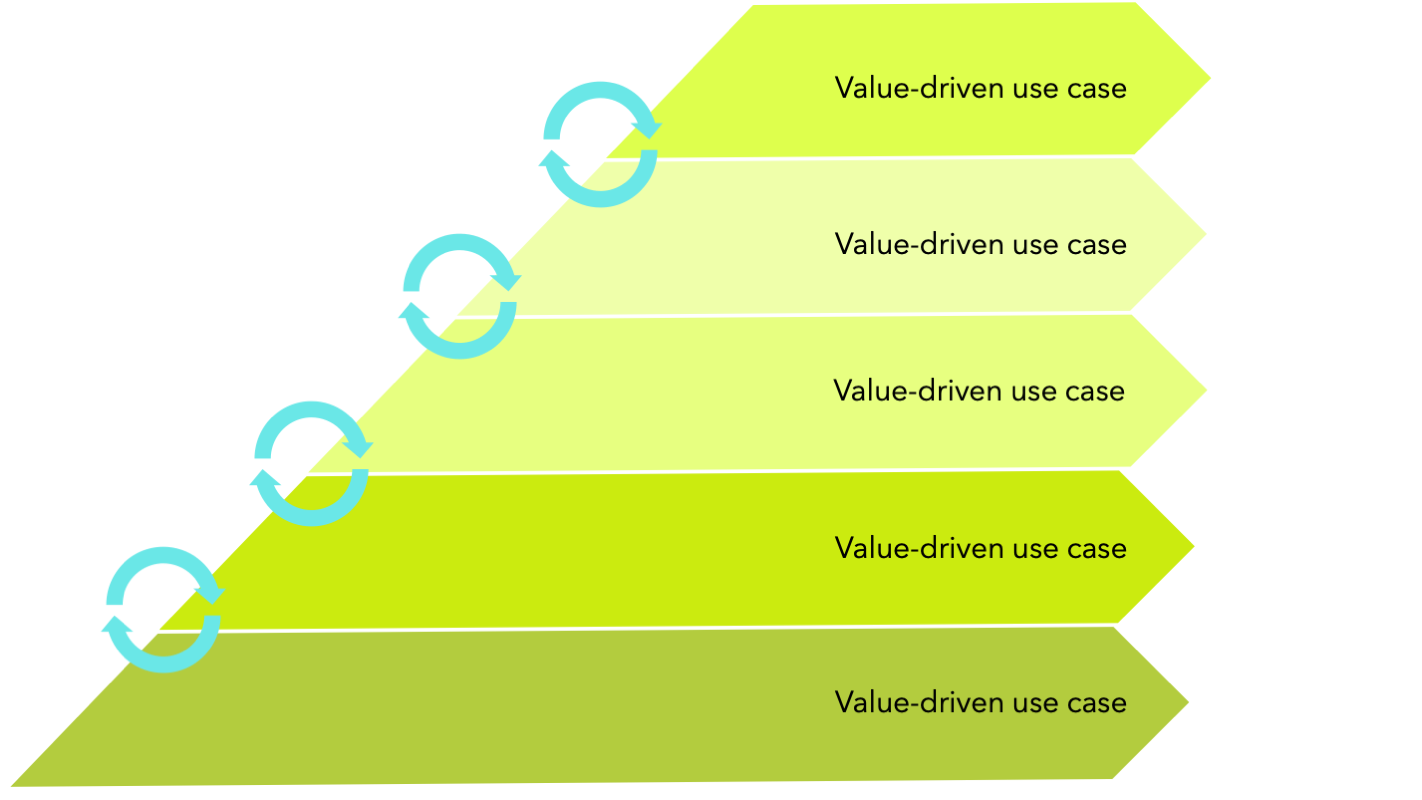
AIOps addresses three key aspects of AI product management, all of which are central to maintaining and optimizing AI systems over time:
- Testing and validation, deployment, and continuous optimization: Before AI systems are deployed, rigorous testing and statistical validation ensure that models are reliable, accurate, and aligned with business objectives. This phase includes defining success measures, validating models against real-world data, and conducting controlled experiments to assess performance. Once deployed, AIOps facilitates continuous optimization, iterating on models and adapting them to fit evolving business goals and requirements. Continuous optimization is essential for maintaining AI relevance and performance in dynamic business environments.
- Monitoring and risk management: AIOps introduces continuous monitoring to validate AI performance and proactively identify areas for improvement. Monitoring includes capturing and storing user interactions with AI outputs, enforcing predefined guardrails, and tracking key performance metrics. Effective monitoring ensures that AI models continue to perform as expected while minimizing risks associated with drift or misalignment with business goals.
- Seamless promotion: AIOps also facilitates the seamless promotion of new AI models and versions into production environments. This ensures that new iterations of AI systems are integrated with minimal disruption, allowing teams to deploy updates quickly. The promotion phase includes making the latest AI artifacts and data available for testing and ensuring that user-facing interfaces are updated appropriately.
Integrating these elements with AIOps allows organizations to shift from isolated, siloed AI experimentation to a cohesive, collaborative process that is both faster and more strategic. By focusing on business-oriented ideation, AIOps aligns technical teams and business stakeholders to create solutions that are technically sound and deliver tangible business value. As AIOps matures, it continues to form the solid foundation necessary for future use cases, enabling organizations to address challenges proactively and drive digital transformation.
The downside of delaying AIOps
Without a unified AIOps framework, a familiar pattern emerges: deployment cycles stall, maintenance efforts become unsustainable, and AI experiments either fail to progress beyond the experimental stage or become obsolete by the time they reach production. This rapid obsolescence is increasingly a major issue for companies, as AI models tend to become outdated much faster once in production, creating significant risk. A lack of coordination between teams results in siloed AI experimentation, with little visibility into resource allocation across projects. Limited monitoring hinders the ability to identify issues or make necessary enhancements, while manual or ad hoc deployments of AI models create inefficiencies and inconsistent outcomes. Furthermore, many organizations rely on siloed research and analytics operating models, which hamper collaboration and prevent a cohesive, integrated approach to AI deployment and optimization. Collectively, these barriers impede AI’s full potential, reducing its business impact and the return on AI investments.
To fully realize the AI lifecycle, organizations need a strategic, proactive approach to implementation. This means embracing calculated risks and developing a scalable AIOps framework that transitions AI solutions from experimentation to production while evolving alongside organizational needs. By holistically addressing challenges, this approach accelerates the AI lifecycle, enabling faster deployment and iteration. The resulting speed translates to quicker realization of business value, maximizing ROI on AI investments. This faster, more efficient approach not only helps organizations stay ahead of the competition but also ensures that AI solutions deliver meaningful, lasting impact.
Our approach
At Slalom, we believe that success in AIOps is not just about deploying the right tools but about fostering a holistic, integrated approach that seamlessly fits within existing business operations. Our AIOps framework establishes a solid technology and architecture foundation, guiding organizations from concept to fully tested, reliable technology implementation. This approach ensures alignment across all systems and stakeholders, enabling seamless integration and scalability. To ensure AI/ML operations are successful, we focus on the following key best practices, each of which delivers clear benefits for organizations aiming to maximize their AI capabilities:
- Holistic integration of AI/ML: Successful AI/ML adoption requires seamless integration with existing systems and processes. By aligning AI/ML capabilities with governance and security frameworks from the outset, organizations can accelerate implementation, reduce complexity and risk, and improve time to market for AI solutions. This approach ensures that AI models are deployed efficiently and aligned with business goals, enabling faster speed to market.
- Prioritizing user experience: The ultimate value of AI/ML models lies in their adoption by end users. Involving UI/UX experts early in the process ensures that AI solutions are not only accurate but also intuitive, user-friendly, and aligned with business needs. By prioritizing user experience, organizations can increase adoption rates and overall project success, which directly enhances customer satisfaction through personalization and boosts business outcomes.
- Efficient AI operations: Efficiency throughout the AI lifecycle—from data preparation to deployment, monitoring, and ongoing validation—is critical to achieving faster time to value. Streamlining operations through automation and process optimization reduces costs while delivering better outcomes. By leveraging automation and architectural best practices, we ensure that AI/ML models are efficiently deployed and optimized, minimizing errors and maximizing performance. Automating key processes like model deployment and monitoring allows businesses to quickly transition from experimentation to production, accelerating the realization of measurable ROI.
- Designing for scalability: AI/ML systems must be designed with scalability in mind to meet evolving organizational needs. Scalable solutions that operate across platforms and environments ensure that businesses continue to derive value as their requirements expand. This flexibility enables organizations to adapt to market demands and maintain operational agility, ensuring long-term success through efficient collaboration and seamless integration of AI systems.
By embedding these principles within a holistic AIOps framework, organizations can accelerate time to market, enhance collaboration between teams, and ensure AI systems scale seamlessly. As a result, businesses can drive more meaningful transformation, unlock continuous innovation, and achieve lasting benefits such as improved efficiency, reduced costs, and greater return on investment.
Building for the future
As AI solidifies its position as a cornerstone technology, AIOps is more critical than ever. Organizations must transition from viewing AI as departmental experiments to treating it as a core business competency. This shift demands moving beyond ad hoc methods to embrace operational best practices, ensuring efficient and systematic AI investments.
A holistic, human-centered approach to operationalizing AI/ML helps organizations navigate the complexities of the AI lifecycle. This strategy ensures AI initiatives are not only successful but also sustainable in delivering long-term business value. AIOps plays a crucial role in this transition, helping businesses accelerate their AI journey from POC to full-scale production. By adopting AIOps, organizations can maximize both short-term wins and long-term success, moving decisively beyond experimentation to achieving real-world success.